Lecture 07: Locality of Reference
COSC 273: Parallel and Distributed Computing
Spring 2023
Coming Soon!
- Lab 02: Computing Shortcuts
- HPC cluster instructions
Outline
- Activity: Locality of Reference
- download
lec07-locality-of-reference.zip
from website
- Computer Architecture, Oversimplified
- Computing Shortcuts
Two Stories
- Multithreaded performance
- embarrassingly parallel computaton
- e.g., estimating $\pi$
- Multithreaded correctness
- e.g.,
Counter
example
- mutual exclusion (continued next week)
Today:
- Single-threaded performance!
- locality of reference
LocalAdder.java
LocalAdder
Class
Task. Create an array of random (float
) values and compute their sum.
Two Solutions.
- Sum elements in sequential (linear) order
linearIndex = [0, 1,...,size-1]
- Sum element in random order
-
randomIndex
stores shuffled indices
Two Implementations
Linear Sum:
float total = 0;
for (int i = 0; i < size; ++i) {
int idx = linearIndex[i];
total += values[idx];
}
return total;
Random Sum:
float total = 0;
for (int i = 0; i < size; ++i) {
int idx = randomIndex[i];
total += values[idx];
}
return total;
Tester
AdderTester
:
- computes linear sum
- computes random sum
- compares running times
Parameters:
-
STEP
the step size been array tests
-
START
starting size value
-
MAX
maximum size value
Activity
Run AdderTester
for a wide range of sizes:
- 1,000 – 10,000
- 10,000 – 100,000
- 100,000 – 1,000,000
- 1,0000,000 – 10,000,000
- 10,000,000 – 100,000,000
Questions.
-
How do running times compare between linear/random access for smaller arrays? What about larger arrays?
-
How does running time scale with linear/random access?
-
Did you expect to see the trend you see?
How do running times compare?
Can you explain the trend?
Architecture, Less Oversimplified
Idealized Picture
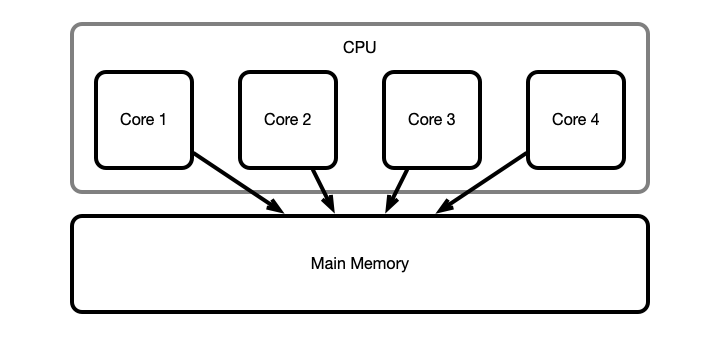
Unfortunately
Computer architechture is not so simple!
- Accessing main memory (RAM) directly is costly
- ~100 CPU cycles to read/write a value!
- Use hierarchy of smaller, faster memory locations:
- caching
- different levels of cache: L1, L2, L3
- cache memory integrated into CPU $\implies$ faster access
A More Accurate Picture
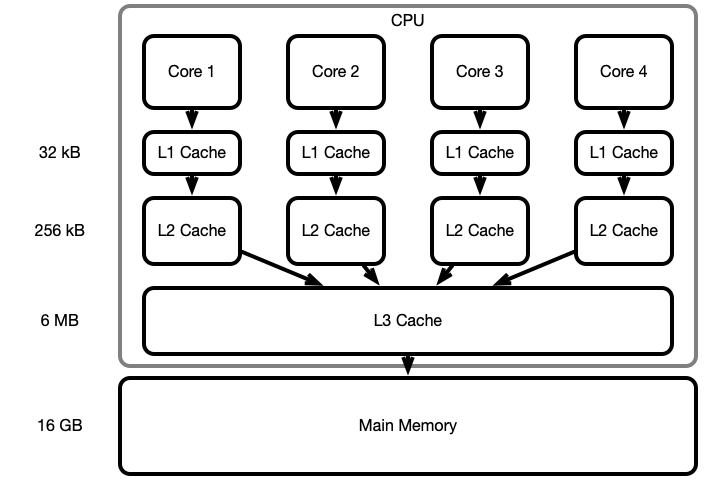
How Memory is Accessed
When reading or writing:
- Look for symbol (variable) successively deeper memory locations
- Fetch symbol/value into L1 cache and do manipulations here
- When a cache becomes full, push its contents to a deeper level
- Periodically push changes down the heirarchy
Memory Access Illustrated
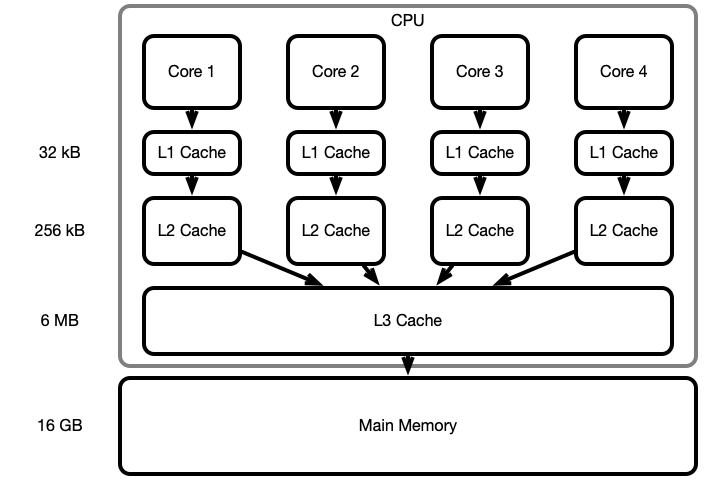
Why Is Caching Done? Efficiency!
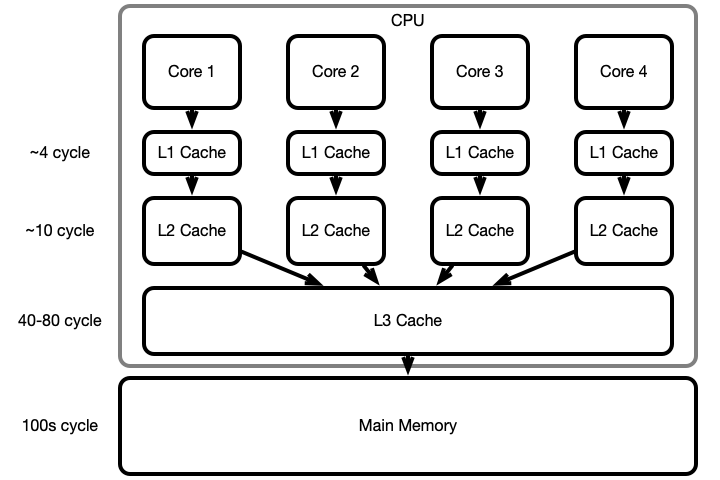
Why Caching Is Efficient
Heuristic:
- Most programs read/write to a relatively small number of memory locations often
- These values remain in low levels of the hierarchy
- Most commonly performed operation are performed efficiently
Why Caching is Problematic
Cache (in)consistency
- L1, L2 cache for each core
- Multiple cores modify same variable concurrently
- Only version stored in local cache modified quickly
- Same variable has multiple values simultaneously!
Takes time to propogate changes to values
- Shared changes only occur periodically!
What Your Computer (Probably) Does
arr
a large array
On read/write arr[i]
, search for arr[i]
successively in
- L1 cache
- L2 cache
- L3 cache
- main memory
Copy arr[i]
and surrounding values to L1 cache
- usually
arr[i-a],...,arr[i+a]
ends up in L1
This process is called paging
Lab 02: Computing Shortucts
A Network
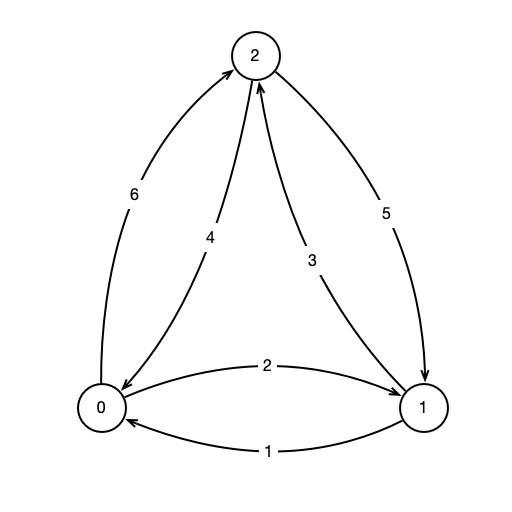
Network
-
nodes and edges between nodes
- nodes labeled $0, 1, \ldots, n$
-
directed edges $(i, j)$ from $i$ to $j$ for each $i \neq j$
- edges $(i, j)$ have associated weight, $w(i, j) \geq 0$
- weight indicates cost or distance to move from $i$ to $j$
Shortcuts
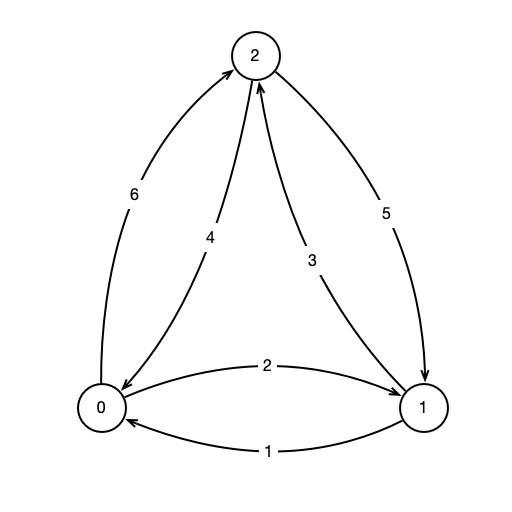
What is cheapest path from 0 to 2?
A Problem
Given a network as above, for all $i \neq j$, find cheapest path of length (at most) 2 from $i$ to $j$
- weight of a path is sum of weight of edges
- convention: $w(i, i) = 0$
- a shortcut from $i$ to $j$ is a path $i \to k \to j$ where $w(i, k) + w(k, j) < w(i, j)$
Shortcut Distances
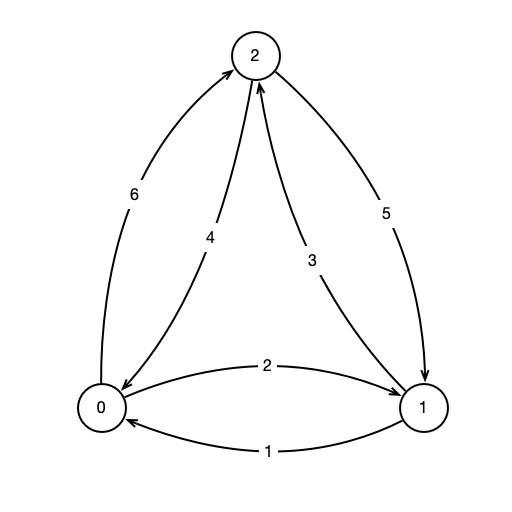
Computing Output
- $D = (d_{ij})$
- Output $R = (r_{ij})$
- $r_{ij}$ = shortcut distance from $i$ to $j$
- computed by \(r_{ij} = \min_k d_{ik} + d_{kj}\)
Example
-
\[D =
\left(
\begin{array}{ccc}
0 & 2 & 6\\
1 & 0 & 3\\
4 & 5 & 0
\end{array}
\right)\]
-
\[R =
\left(
\begin{array}{ccc}
0 & 2 & 5\\
1 & 0 & 3\\
4 & 5 & 0
\end{array}
\right).\]
In Code
- Create a
SquareMatrix
object
-
SquareMatrix
stores a 2d array of float
s called matrix
-
matrix[i][j]
stores \(w(i, j)\)
Your Assignment
Write a program that computes shortcut matrix as quickly as possible!
- You’ll be given
getShortcutMatrixBaseline()
- Your assignment is to optimize the code to write
getShortcutMatrixOptimized ()
Assignment Challenges
- Optimize memory access pattern for operations
- make access pattern linear, when possible
- Apply multithreading to get further speedup
- partition the problem into smaller parts
Payoff: optimized program will be 10s of times faster on your computer, 100s of times faster on HPC cluster!